AI Enhances Precision in Routine Medical Testing
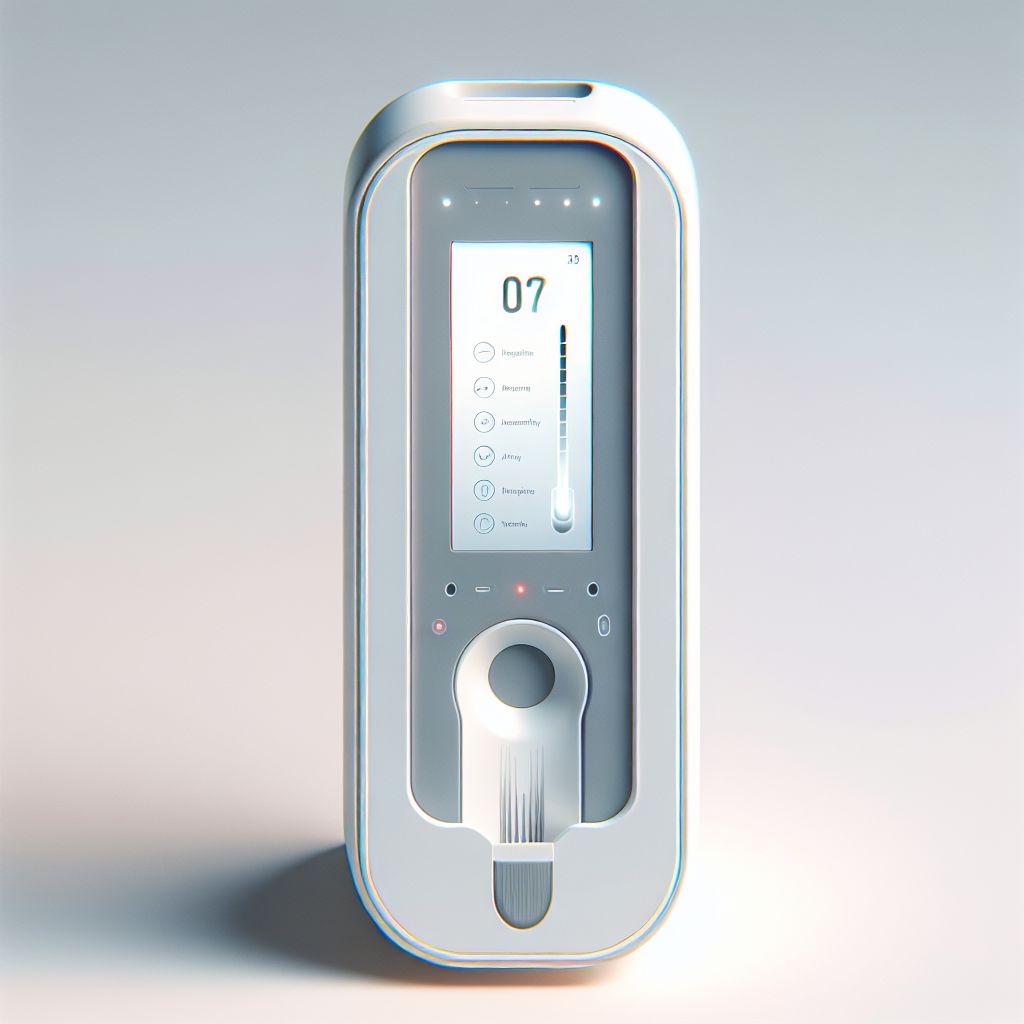
Introduction to Complete Blood Counts
Complete Blood Count (CBC) tests are one of the most commonly performed blood tests worldwide, running billions of times each year. These tests provide a detailed profile of your blood cells, including red blood cells, platelets, and white blood cells. Doctors use CBC tests to diagnose various conditions and monitor overall health. Whether you're having an annual physical or being evaluated for a specific health issue, a CBC test is often part of the routine process.
Current Challenges with CBC Interpretation
Despite their widespread use, interpreting CBC results can be less precise than ideal. Currently, clinicians rely on standard reference intervals that apply to the general population. These one-size-fits-all ranges do not account for individual differences in genetics and environment. As a result, what is considered normal for one person may not be normal for another, potentially leading to misdiagnoses or unnecessary further testing.
Advancements in Personalized Blood Test Analysis
Researchers at the University of Washington School of Medicine, working alongside the Higgins Lab at Harvard Medical School, are pioneering the use of computational tools to enhance CBC interpretation. By analyzing 20 years of blood count data from tens of thousands of patients across the East and West coasts, they have developed machine learning models to determine individual 'set points' for blood count markers. This personalized approach aims to redefine what 'normal' means for each patient, improving diagnostic accuracy and patient care.
Understanding Blood Markers and Their Significance
CBC tests measure various blood cell types, each indicating different aspects of your health. Hemoglobin levels, for example, reveal how well your blood carries oxygen, while platelet counts indicate your blood's ability to clot and stop bleeding. White blood cell counts reflect your immune system's activity. Changes in these markers can signal conditions like iron deficiency, infections, or internal bleeding. Understanding these markers helps doctors diagnose and monitor a wide range of health issues.
Benefits of Personalized Reference Ranges
By establishing individual normal ranges, researchers found that personal set points are typically narrower than population-based ranges. For instance, while the general normal range for white blood cells is 4.0 to 11.0 billion cells per liter, an individual’s range might be 4.5 to 7 or 7.5 to 10. Using these personalized ranges allows for more accurate detection of abnormalities, leading to better diagnosis of diseases like iron deficiency and chronic kidney disease. Additionally, these set points can predict future health risks, such as the likelihood of developing Type 2 diabetes.
Future Implications for Personalized Medicine
The integration of personalized blood count set points into clinical practice holds significant promise for the future of medicine. Doctors could use these individualized ranges to enhance disease screening and interpret test results more accurately. This approach represents a shift towards personalized medicine, where each patient's unique medical history and biological makeup inform their healthcare. Ultimately, this could lead to more effective treatments and better health outcomes tailored to each individual.
Conclusion
Complete Blood Count tests are essential tools in modern medicine, but their effectiveness can be limited by broad reference ranges. The innovative research conducted by mathematicians and medical scientists is paving the way for more personalized and precise blood test interpretations. By leveraging machine learning and extensive patient data, it's now possible to define individual normal ranges, enhancing diagnostic accuracy and predicting future health risks. This advancement marks a significant step forward in personalized medicine, promising improved patient care and better health outcomes for individuals.
Read the full article here:
inverse.com